Explore NanoString GeoMx DSP Spatial Transcriptomics with BBrowser
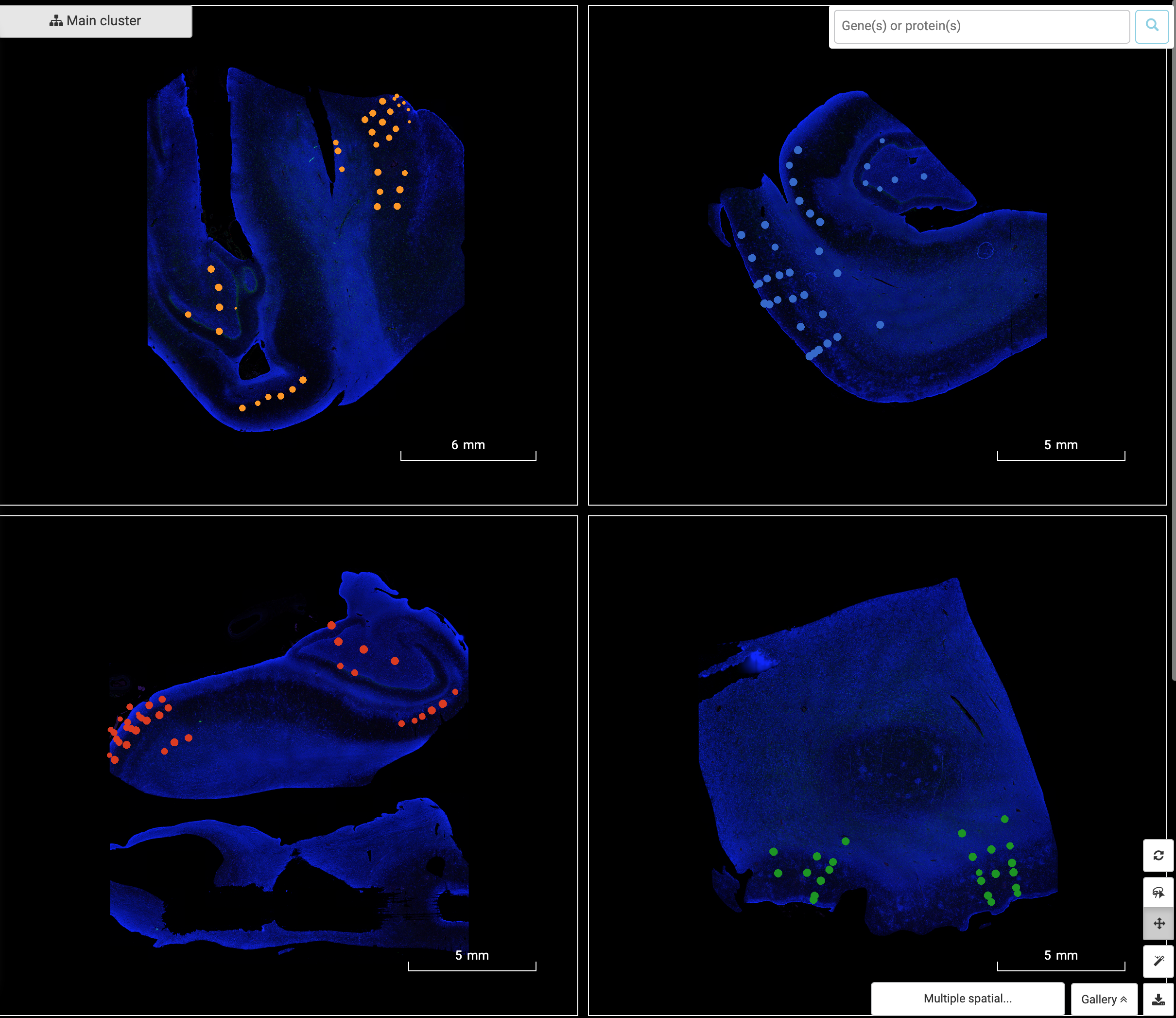
From Single-cell RNA-sequencing to Spatial RNA-sequencing Since its invention, single-cell RNA-sequencing has become one of the most popular genomic tools. Despite its power in dissecting the transcriptome at individual cell resolution, single-cell RNA-sequencing stil faces several limitations. One of them is the lack of critical spatial information. Adding spatial context […]